Keynote Speakers
We are very pleased to have acquired the services of excellent keynote speakers for the conference. The speakers and the titles of their talks are shown below.
Prof Takushi Tanaka
Fukuoka Institute of TechnologyJapan
Handmade Satellite NIWAKA (FITSAT-1)
More details ...
Prof Andrzej Skowron
Warsaw UniversityPoland
Rough Sets and Reasoning about Complex Vague Concepts
More details ...
Prof Chen-Fu Chien
National Tsing Hua UniversityTaiwan
Manufacturing Intelligence and Data Mining: From Yield Enhancement, Production Management, to Manufacturing Strategy in Semiconductor Manufacturing
More details ...
Prof Mika Sato-Ilic
University of TsukubaJapan
Cluster-based Scaling for Symbolic Data and its Applications in Decision Making
More details ...
Other speaker's details to follow ...
Prof Takushi Tanaka
Fukuoka Institute of TechnologyJapan
Handmade Satellite NIWAKA (FITSAT-1)
Abstract:
We have developed a small artificial satellite named NIWAKA (FITSAT-1). The shape is a 10cm cube, and the weight is 1.33kg. The main mission of this satellite is to demonstrate the high speed transmitter developed (5.84GHz, 115.2kbps). It can send a jpeg VGA-picture (480x640pixels) within 6 seconds. NIWAKA has another experimental mission to test the possibility of optical communication by satellite. It will actually twinkle as an artificial star. NIWAKA will be launched from the International Space Station around September 2012.
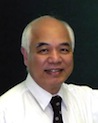
Biography:
Takushi Tanaka received the B.Eng., M.Eng., and Dr.Eng degrees from Kyushu University, Fukuoka, Japan, in 1967, 1969, and 1987, respectively. From 1982 to 1983, he was a Post-Doctoral Fellow with the Department of Computer Science, Yale University, where he was involved with the AI-project.
From 1976 to 1988, he worked on understanding natural language as a Senior Researcher at The National Language Research Institute, Tokyo, Japan.
He is currently a Professor with the Department of Computer Science and Engineering at the Fukuoka Institute of Technology since 1988.
His research interests include Artificial Intelligence, Language Processing, Logic Programming, Modeling Knowledge for Electronic Circuits, Robot Soccer, and Cube-sat Artificial Satellite.
Prof Andrzej Skowron
Warsaw UniversityPoland
Rough Sets and Reasoning about Complex Vague Concepts
Abstract:
Vagueness is not allowed in mathematics, interesting for philosophy, and is a nettlesome problem in natural language, cognitive science, machine learning, data mining, and, in general, in artificial intelligence and computer science. For example, in robotics or multiagent systems complex vague concepts may concern, e.g., risk, social issues or emotions. Judgment strategies are used for estimation of truth degrees of sentences such as object x belongs to the vague concept C. We discuss Interactive Rough Granular Computing (IRGC) and applications of IRGC to approximation of vague concepts and approximate reasoning about such concepts. Approximation of complex vague concepts can be supported by domain knowledge, in particular by ontologies. We present illustrative applications of approximation of complex vague concepts and approximation of domain ontologies in real-life projects related to medical decision support, identification of complex behavioral patterns by UAV, sunspot classification, and dialog based search engines. Moreover, we present our recent results on reasoning about complex vague concepts. In particular, we discuss method for reasoning about changes and applications to financial data analysis.
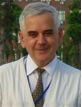
Biography:
Andrzej Skowron received the Ph. D. and D. Sci. (habilitation) from the University of Warsaw in Poland. In 1991 he received the Scientific Title of Professor. He is Full Professor in the Faculty of Mathematics, Computer Science and Mechanics at Warsaw University. Andrzej Skowron is the (co)author of more than 350 scientific publications. His areas of expertise include reasoning with incomplete information, approximate reasoning, soft computing methods and applications, rough sets, rough mereology, granular computing, synthesis and analysis of complex objects, intelligent systems, knowledge discovery and data mining, decision support systems, adaptive and autonomous systems, perception based computing. He was the supervisor of more than 20 PhD Thesis. In the period 1995-2009 he was the Editor-in-Chief of Fundamenta Informaticae journal. He is on Editorial Boards of many others journals. Andrzej Skowron was the President of the International Rough Set Society from 1996 to 2000. He has delivered numerous invited talks at international conferences including plenary talk at the 16-th IFIP World Computer Congress (Beijing, 2000), keynote talk at 8th Joint Conference on Information Sciences (JCIS 2005) (encompassing 12 individual conferences and workshops) (USA, 2005), invited talk at 2006 IEEE/WIC/ACM International Conference on Intelligent Agent Technology (IAT 2006) and on Web Intelligence (WI 2006) (Hong Kong, 2006), and plenary talk at the 2-nd World Congress on Biologically Inspired Computing (Japan, 2010).
Prof Chen-Fu Chien
National Tsing Hua UniversityTaiwan
Manufacturing Intelligence and Data Mining: From Yield Enhancement, Production Management, to Manufacturing Strategy in Semiconductor Manufacturing
Abstract:
This keynote aims to address emerging research issues and challenges driven by the needs of semiconductor manufacturing that is highly automated and integrated to enhance the decision quality and speed of the decisions involved in yield enhancement at the engineering level, production management in operational level, and manufacturing strategy in corporate level. Based on my extensive collaborative studies with semiconductor manufacturing companies and the trends I have observed in the past few years, this talk will review a number of research and case studies in different levels to illustrate the thoughts and lessons learned to inspire more discussions in research communities. Indeed, semiconductor manufacturing is one of the most capital-intensive and fast clock-speed industries that is driven by Moore's Law for continuous improvements for technology advance and cost reduction. Similar advanced equipment and processes are applied to produce a variety of semiconductor products including microprocessors, memories, digital signal processor, and application-specific logic. Many semiconductor companies compete on selling interchangeable products or providing foundry services with similar process technologies to customers worldwide. As moving into consumer electronics era, semiconductor products have increasingly shortening product life cycles with demand fluctuation. Therefore, high-tech companies are confronting with various decision problems involved in strategy, manufacturing, and technology that are characterized by uncertain (incomplete or massive) information and a need for tradeoff among different objectives and justification for the decisions to align with the overall strategic objectives. Indeed, we proposed a corporate manufacturing strategic decision framework, PDCCCR, that integrated the interrelated elements including pricing strategies (P), demand forecast and demand fulfillment planning (D), capacity planning and capacity portfolio (C), capital expenditure (C), and cost structure (C), that will affect the overall return (R) of a company. Furthermore, manufacturing intelligence (MI) and data mining approaches that are enabled by the fully automation high-tech manufacturing facilities have been developed to model the right decision problems and extract useful information to estimate the parameters and derive the intelligence to enhance decision quality effectively and efficiently. Finally, we conclude this talk with discussion of the ongoing changes and opportunities of manufacturing intelligence and data mining in semiconductor manufacturing.
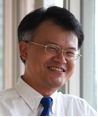
Biography:
Chen-Fu Chien is a Distinguished Professor in National Tsing Hua University (NTHU), Taiwan. He has been with the Department of Industrial Engineering and Engineering Management, NTHU since 1996. He is the Director of Science Park Sustainable Growth Promotion Project Office of National Science Council (NSC), Taiwan to sponsor university-industry collaborative research to enhance the competence during the financial tsunami. He is also a program leader for industrialization and virtual integration for the National Project of Intelligent Electronics from 20011 to 2015. He received B.S. (with Phi Tao Phi Honor) with double majors in Industrial Engineering and Electrical Engineering from NTHU in 1990. He received M.S. of Industrial Engineering and Ph.D. of Operations Research and Decision Sciences from UW-Madison in 1994 and 1996, respectively. He was a Fulbright Scholar in IEOR, UC Berkeley from 2002 to 2003 and also received the Executive Training of PCMPCL in Harvard Business School in 2007. From 2005 to 2008, he had been on-leave to serve as the Deputy Director of Industrial Engineering Division in Taiwan Semiconductor Manufacturing Company (TSMC), which is the world largest semiconductor foundry.
Since 2002, Dr. Chien has been a Steering Committee Member of Industrial Engineering and Management Division in NSC, Taiwan. He is a Think Tank member for Government Science and Technology Projects in National Science Council and also on the Board of Directors of the Chinese Institute of Industrial Engineers (CIIE) and Chinese Institute of Decision Sciences in Taiwan. He had also been an Adjunct Professor of Tianjin University and Visiting Professor at the Cambridge University (sponsored by Royal Society), Beijing Tsinghua University (sponsored by Chinese Development Foundation), and Waseda University (sponsored by Japan Interchange Association Young Scholar Fellowship). He is now an Associate Editor of IEEE Transactions on Automation Science and Engineering, Area Editor of Flexible Services and Manufacturing Journal, and an Advisory Board Member of OR Spectrum. He had been a guest editor for leading journals including IEEE Transactions on Automation Science and Engineering, OR Spectrum, IJPE, C&IE, JIM.
His research efforts center on decision analysis, modeling and analysis for semiconductor manufacturing, manufacturing strategy, and data mining. Dr. Chien has received seven international invention patents on semiconductor manufacturing and published two books, more than 80 journal papers and a number of case studies in Harvard Business School. He has been invited to give keynote and plenary talk in various conferences such as APIEMS, C&IE, IEEM, IML and leading universities including Harvard Business School, Cambridge University, UC Berkeley, Waseda University, and IBM Watson Research Center. His keynote speech in IEEM 2006 was then invited by IIE to publish as a feature article in Industrial Engineer (2007 Feb, pp. 47-49). Dr. Chien has received many awards including Outstanding Research Awards (2007, 2011), Tier-One Principal Investigator (2005-2008), and Best Research Awards from the National Science Council, University Industrial Contribution Award from Ministry of Economic Affairs (2009, 2010), Distinguished University-Industry Collaborative Research Award from the Ministry of Education (2001), Distinguished Young Faculty Research Award (2001) and Distinguished University-Industry Collaborative Research Award (2007) by NTHU, Best Paper Award (2001) and Distinguished Young Industrial Engineer Award (2001) from Chinese Institute of Industrial Engineers, Best Engineering Paper Award (2002) and Distinguished Engineering Professor (2010) by Chinese Institute of Engineers, TSMC-NTHU Faculty Semiconductor Research Grant (2004), and the Lu, Feng-Chang Award from Chinese Management Association (2007).
Prof Mika Sato-Ilic
University of TsukubaJapan
Cluster-based Scaling for Symbolic Data and its Applications in Decision Making
Abstract:
Recently there are large and complex data sets challenging the engineering and statistical research world. The complexity is due to four characteristics of the data. First is the status of measurement error caused by noise or incomplete data. Second are the data types and features such as high dimension low sample-size data and multi-mode multi-way data. Third is uncertainty which is generally treated as fuzzy, functional, modal, or interval-data among others. Fourth is the big data itself which is passively involved in the complexity of the data. I will present several methods of Cluster-based scaling to deal with the large and complex data.
We proposed Cluster-based scaling which obtains the latent structure of data in the reduced lower dimensional space through the intervening solution space of the clustering result. We have also exploited symbolic data analysis to deal with the complexity of the data within Cluster-based scaling. Symbolic data analysis is based on knowledge discovery which is included in computational statistics. Symbolic data analysis is targeted at the conceptual world, so the data has a systematic hierarchy. Obtaining this systematic hierarchy is essentially the guiding principal of clustering methods. This is the reason we use this analysis for the cluster-based scaling.
Several case studies that have applied the proposed cluster-based scaling will be presented.
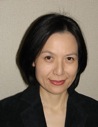
Biography:
Prof. Mika Sato-Ilic received a Doctorate of Engineering from Hokkaido University, specializing in Intelligent Data Analysis. She currently holds the position of Associate Professor in the Faculty of Engineering, Information and Systems, at the University of Tsukuba, Japan.
She is the founding editor-in-chief of the International Journal of Knowledge Engineering and Soft Data Paradigms, Associate Editor of Neurocomputing, and Associate Editor of the International Journal of Innovative Computing, Information and Control Express Letters, as well as serving on the editorial board of several other journals. In addition, she is currently a council member of the International Association for Statistical Computing which is a section of the International Statistical Institute (ISI) and a Senior Member of the IEEE where she holds several positions including the Vice-Chair of the Fuzzy Systems Technical Committee of the IEEE Computational Intelligence Society, and a member of the IEEE Women in Computational Intelligence Committee. In addition, she has served on several IEEE committees including the administration committee, vice program chair, special sessions co-chair.
Her academic output includes 4 books, 5 book chapters and over 100 journal and conference papers. Her research interests include the development of methods for data mining, multi-dimensional data analysis, multi-mode multi-way data theory, pattern classification, and computational intelligence techniques for which she has received several academic awards.